Advantage Horizon: Data Analytics Offers an Information Edge
In the Army’s march toward the secretary of the Army’s number two priority, becoming more “data-centric,” Army leaders need more useful models that will help them think about achieving and maintaining an information advantage through data analytics.
Current models, such as the Analytics Journey, show increasing competitiveness as analytic capabilities mature. However, these models are flawed. They are too linear and fail to account for competitive actors that prevent adversaries from dominating an environment. As analytic models mature, competitors do not passively watch as their advantage wanes. Instead, competitors act to disrupt their adversary’s advantage by changing behavior and adjusting the operating environment, driving the adversary’s predictions into disorder and denying future advantage.
The Advantage Horizon model is a better approach, which fits the Analytics Journey to the Cynefin framework. The Advantage Horizon model overcomes the disadvantages of the Analytics Journey because it accounts for competitive environments and highlights the point at which leaders can expect their advantage to wane if left unprotected. The Advantage Horizon is the inflection point when a competitive analytics advantage begins to erode due to competitors taking losses, noticing those losses, beginning to identify advantage and then adapting their behavior to avoid irrelevance. Using this model, Army leaders can evaluate the appropriate use of data models over time, guide analysts to derive useful insights for data-driven decisions and extend their competitive advantage over adversaries.
The Analytics Journey models how organizations evolve their use of data analytics over time. It reflects the process that data analysts follow to develop models that increase in value as they mature. The process begins with descriptive analytics to recognize what is happening. Next, analysts use exploratory analysis to identify correlation and causal relationships to infer why events occur. Once analysts understand why events occur, they use predictive analytics, such as linear regression, to estimate the likelihood of future events occurring. Finally, as the model matures and its accuracy improves, analysts develop best practices that prescribe actions based on sensed data. As analysts progress through the four stages of the analytics journey, they support better situational understanding and improved decision-making, which the model incorrectly associates with an increased competitive advantage.
The problem with the Analytics Journey model is that it does not consider competitive and adaptive adversaries. At each step of the analytics journey, adversaries will adapt their behavior to avoid being outmaneuvered. They will seek to minimize the Army’s advantage by stealing intellectual property, engaging in deceit or changing behavior in unpredictable ways. Army leaders must protect their gained advantage by erecting barriers, employing deception and implementing effective information security measures. Leaders who leave their advantage unprotected will lose it.
A better way for leaders to view the analytics journey in a competitive environment is through the Advantage Horizon model. This model fits the Analytic Journey to the Cynefin framework and highlights the point at which an unprotected competitive advantage wanes. A leader’s objective is to protect and extend their competitive advantage and prevent being driven into disorder. The Advantage Horizon is the inflection point when a competitive analytics advantage begins to erode. At this inflection point, competitors and adversaries begin taking losses due to the competitive advantage. They notice these losses and begin taking steps to identify the advantage and adapt their behavior to avoid irrelevance. Additionally, the model allows for temporary reversal of gains, as illustrated by the dashed lines, and highlights the possibility of additional maturity not being possible as leaders and analysts cycle between stages in the analytics journey.
As leaders use data to build models that improve decision-making, they must take steps to protect and extend their Advantage Horizon. Business leaders extend their Advantage Horizon using intellectual property protections, such as patents, copyrights and trademarks, or by protecting trade secrets with nondisclosure agreements. Military leaders extend their advantage horizon by controlling new knowledge as protected state secrets, employing deception, using sanctions to deny access to key data and technologies or spoiling known data sources to confuse the adversary.
In addition to identifying the Advantage Horizon, this model highlights the ability of analysts and leaders to veer into the disorder context. In disorder, leaders do not understand the proper context for sensemaking and can unknowingly mistake their placement in any of the four contexts. When operating in disorder, leaders and analysts apply analytical models to nonapplicable environments and draw incorrect conclusions from the results.
Leaders must avoid disorder for themselves while increasing the likelihood that their adversary falls into disorder. One of the most important things a leader can do is to increase their data literacy. Increased data literacy can avoid common errors such as selection bias in data collection, using invalid statistical methods to draw conclusions about an environment or misdiagnosing cause-and-effect relationships. Other errors include applying data models and algorithms to ill-suited environments, becoming complacent with the environment and ignoring changes that invalidate a model’s predictive capabilities. To drive an adversary or competitor into disorder, leaders should spoil data sets and collection, increase complacency, or change behavior to be less predictive.
The Cynefin framework is a leadership decision framework that provides leaders a tool to identify how they should react to issues depending on which of the four contexts they are located: Chaotic, complex, complicated or simple. The authors of the Cynefin framework offer a fifth context, disorder, which applies when a predominate context is not discernible. The four stages of the Analytics Journey model can be fitted to the four contexts offered in the Cynefin framework to understand how a data-analyst approach to analytic modeling changes throughout their journey.
In the chaotic context, leaders should act-sense-respond to situations. This context shares similarities with data discovery in the Analyst Journey. When an analyst first gathers data for exploratory analysis, they have no idea how to describe the environment. Therefore, the analyst must first act by gathering data, sense through analysis and then respond by deciding if the data sufficiently describes the environment, or if more data are needed.
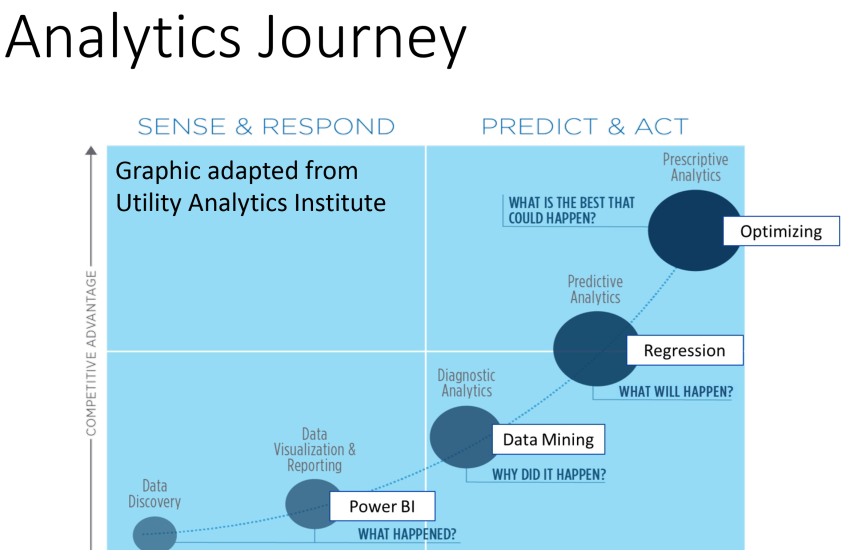
Once enough data are gathered for leaders to visualize the environment, the analyst moves into the complex context, where leaders should probe-sense-respond to situations. Similarly, analysts conduct causal analysis in the same manner as hypothesis testing. They probe the environment by making controlled changes; they sense if the change had its intended effect and then decide if the causal relationship is strong enough to determine why a situation happened; if not, they probe and consider additional variables.
However, if the causal relationship is strong enough to be a good predictor of future outcomes, the analyst moves to the complicated context. In this context, where leaders should sense-analyze-respond to situations, the analyst uses their algorithm as an expert system to sense the data in the current environment, analyze with predictive analytics and make probability-based recommendations to leaders. When their algorithm can predict outcomes with near certainty, an analyst moves to the simple context. In this context, the analyst’s algorithm senses data in the environment, categorizes it as a known issue and then responds with the intended action.
Operation Ultra provides an illustrative example of British and German leaders developing an advantage through analytics and then extending their advantage horizons. Operation Ultra was the British intelligence program to intercept and decipher German communications encrypted with the Enigma Machine during World War II. Germany developed the Enigma Machine to encrypt wireless and cable messages. To sustain this advantage, the German military used water-dissolvable paper to distribute daily enigma settings, sent fake message traffic to disorient crypto analysts and improved the enigma’s cryptographic complexity as the war went on.
Combating this advantage in cryptographic technology, the allied forces began by collecting data through signals intercepts, document exploitation from captured German units and old-fashioned espionage techniques. They painstakingly analyzed the data, eventually discovering how the Enigma Machine encoded messages. This discovery allowed allied forces to intercept German messages, decrypt war plans and predict German military actions. Through closely held secrets and deception, the allied forces kept their knowledge of the Enigma codes hidden from the Germans. Unaware that their communications were compromised, the Germans had no reason to adapt their tactics. On several occasions, the British intercepted German submarine positions but would then fly a spotter aircraft over the positions to make the Germans think the aircraft was the source of the knowledge. Even as the war ended, the German military were still under the misconception that the allied forces could not break their enigma encryption.
Additionally, Operation Ultra provides a good example of the German’s cycling in and out of the disorder context because of complacency, and the British experiencing periods of fleeting competitive advantage as they cycle between the complex and complicated contexts. Since the Germans changed Enigma settings daily, the British had to crack the code each morning; some days they succeeded, and some days they did not. On the frequent occasions that the British were able to crack the daily code, the German military unknowingly operated in the disorder context, thinking their plans were spoiled by other means than their communication intercepts. For a short time, the British operated in the complicated context, sensing German transmissions, analyzing them against the decipher code, and then responding to the threat. The Germans’ complacent behavior made the British crypto analysts’ job easier because units frequently sent the same status messages each morning, or they sent duplicate messages over alternate unencrypted mediums. When this occurred, the Germans’ complacency allowed the British to seize a position of temporary advantage early in the day and sustain that advantage until the Germans changed their Enigma settings at the end of the day. Once Enigma settings were changed, the British fell back into the complex context and had to probe-sense-respond as they iterated through decrypting the signals intercepts to discover the new German code and regain their position of advantage.
Leaders seeking to improve decision-making by using data should consider the Advantage Horizon Model to guide them in identifying the point at which they can expect their unprotected competitive advantage to wane. Extending the Advantage Horizon through analytics requires leaders who are data-literate, apply analytic discoveries appropriately and actively protect their advantage while preventing disorder. The Advantage Horizon Model more accurately reflects the competitive advantage derived from maturing analytics and can aid leaders in understanding their role in achieving the secretary’s goal of becoming a more data-centric Army.
Col. Daniel Crawford, USA, is an assistant professor and seminar advisor for the U.S. Army Command and General Staff College’s Information Advantage Scholars program.
The opinions expressed in this article are not to be construed as official or reflecting the views of AFCEA International.
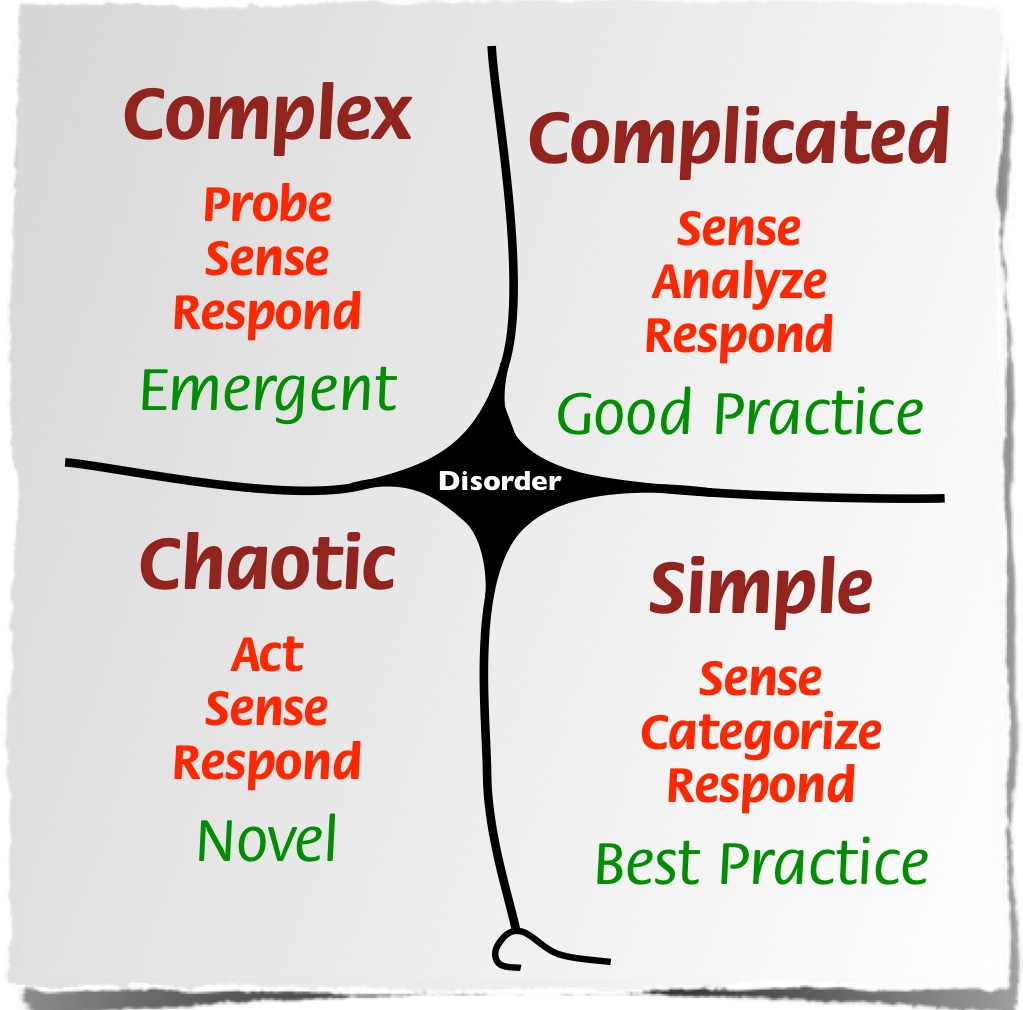
The four stages of the Analytics Journey model can be fitted to the four contexts offered in the Cynefin framework to understand how a data-analyst approach to analytic modeling changes throughout their journey. Graphic by David Snowden