AI as an Insider Threat
Kimberly Underwood’s fine article, “AI Needs of the Air Force,” in the May 2024 edition of SIGNAL Magazine, highlighted the challenges of data management as the U.S. Air Force looks to integrate artificial intelligence (AI) into its operations. In the article, Gen. James C. Slife asked two poignant questions: “Where is the training data?” and “What is your data source?” Reading her article as a cybersecurity professional, my thoughts swirled around the security of this often very sensitive data used by predictive and generative AI models.
The National Institute of Standards (NIST) has published several excellent resources related to the safe development and deployment of AI. Reading through the latest research, one is struck by the apparent disconnect between fear and the real threat. Most concerns revolve around the potential for AI to aid cyber criminals, providing a new and powerful capability for them to automate sophisticated attacks against our systems and infrastructure. However, current research suggests that AI is not yet at a place where it poses this kind of cybersecurity threat. From NIST and others, the real threat posed by AI is the data underlying the model and how it can be manipulated. Many studies confirm that AI tools are not superhuman intellectual machines; they generate output based on the training data, including biases and misinformation.
The published attack vectors for AI are not using AI tools to conduct attacks but poisoning the training data or the model itself, effectively brainwashing the AI. If the attacker can manipulate the training data or the way the model parses it, the attacker can prompt the AI to deliver favorable responses. These responses from the manipulated AI can include delivering false or misleading data, prompting it to reveal sensitive information, or taking destructive action, such as altering settings on an industrial control system or sensor. An additional attack vector does not include changing the AI but exploiting the inherent weakness in how AI processes language and data. This attack involves simply refining prompts that convince the AI to circumvent its programmed responses and do things it was not supposed to do. One writer equated it to a child continuously pestering their parents to buy candy at the grocery store.
Cyber criminals and nation-states have learned that compromising a third-party vendor is often more effective than initiating a direct attack on a protected organization. The SolarWinds hack in 2020 was a perfect example of a supply-chain attack on a third party. By infiltrating SolarWinds’ development chain and implanting malware in a software update, a suspected Russian hacking group was able to deploy malware to thousands of SolarWinds customers, including the federal government. Nation-state actors have posted malicious Docker containers and source code to popular repositories, intending to compromise the work of many application developers. These are deliberately malicious attacks, but there is also the general apathy, ignorance or simple mistakes by legitimate developers and vendors. As AI becomes more ubiquitous, it is important to pay attention to the supply-chain attack vector via third parties. AI must be trained with some data pool acquired from somewhere. While so many are focused on trying to prevent AI-based attacks on their networks, what they really should be focusing on and concerned about is Supply Chain Risk Management (SCRM).
When viewed from a broader perspective, not just that of the Air Force, Gen. Slife’s questions on data sources take on a new urgency. Where is your training data? What is your data source? The questions require that we take a moment to consider a new facet of SCRM as it relates to AI. Not only must we validate the supplier of the AI tool itself, but we must also evaluate the controls they have in place to secure the data and information sources used to train the AI model. Tumblr, Reddit, Shutterstock and WordPress seek to bolster their revenue by selling user data to train AI models. Selling data is big business, and AI training is a new revenue stream for that business. As more brokers enter the market, AI developers must ask themselves if the data is safe, if their data source is reputable and if their model protects sensitive training data. Customers of these AI models must ask the same question.
Vendors for AI and AI-enhanced tools should include a segment in their Systems and Organization Controls 2 (SOC 2), outlining the controls to verify their data sources and protection of their training data. They should also discuss the controls that safeguard their development pipeline to ensure the model isn’t being manipulated to generate favorable output for an attacker. If these questions are not answered in the vendor’s reports, it is imperative that the customer asks these questions, especially if the AI will handle sensitive data or provide input for an important mission or system. If we cannot validate the source(s) and protection of training data or verify the security of the model, that AI tool should be considered unusable. The draft AI Risk Management Framework by NIST addresses some of these questions. The 2024 Report on the Cybersecurity Posture of the United States notes, “As the AI ecosystem continues to evolve, there is an opportunity to ensure that its core elements—data, computing and algorithms—are developed with safeguards against misuse.”
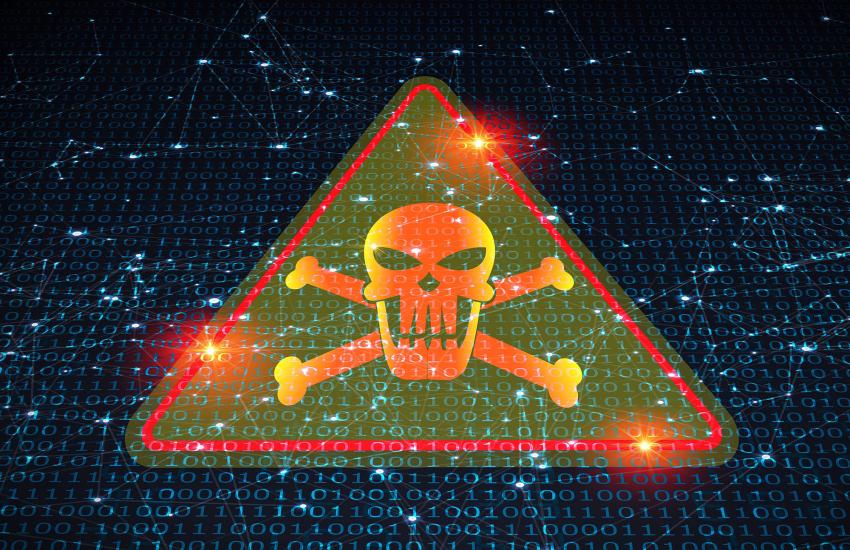
At its current state of development, security controls for AI remain largely in the realm of traditional cybersecurity and secure software development. Developers should ensure only vetted and authorized persons can access the code governing the model and the data that trains it. Activity and changes should be tracked and logged. As training data is cleaned, in addition to finding mistakes, duplicates, incorrect formatting, etc., it should be reviewed for malicious content and its sources verified (perhaps we can learn from the intelligence community, well-practiced at vetting its data sources). Customers should exercise due diligence in their SCRM for acquisition of AI tools. Processes and procedures should be established to flag and report aberrant AI behaviors and responses. Incident Response Plans should include responses to aberrant AI behavior, including procedures for disabling it if necessary.
The war in Ukraine demonstrates how the battlefield domain of cyberspace is growing more central to the art of war. Russia’s hacking teams have proven very effective at manipulating data, sowing dis/misinformation and infiltrating critical infrastructure, including U.S. water supply systems and telecommunications. The National Cybersecurity Strategy calls on government and private sectors to collaborate and cooperate in bolstering our nation’s cybersecurity. AI is only going to grow and become more integrated into our way of life across every industry and, as Kimberly points out, in our armed forces. We must protect our nation’s cybersecurity with the due diligence it deserves. Vendors, use SCRM to verify your data sources. Customers, use SCRM to verify your vendors and their data sources. The insider threat is always your greatest cybersecurity threat, and AI is rapidly becoming an insider.
Shaun Rieth is a 22-year retired Air Force cyber operator who returned to serve as a federal contractor under Management and Engineering Technologies International (METI), currently supporting the 557th Weather Wing on Offutt Air Force Base in Nebraska as a senior cybersecurity analyst in the Defensive Cyber Operations flight. He possesses a Bachelor of Science in IT operations management, an MBA, CCISO and CISSP certifications, and a CCSK from the Cloud Security Alliance.
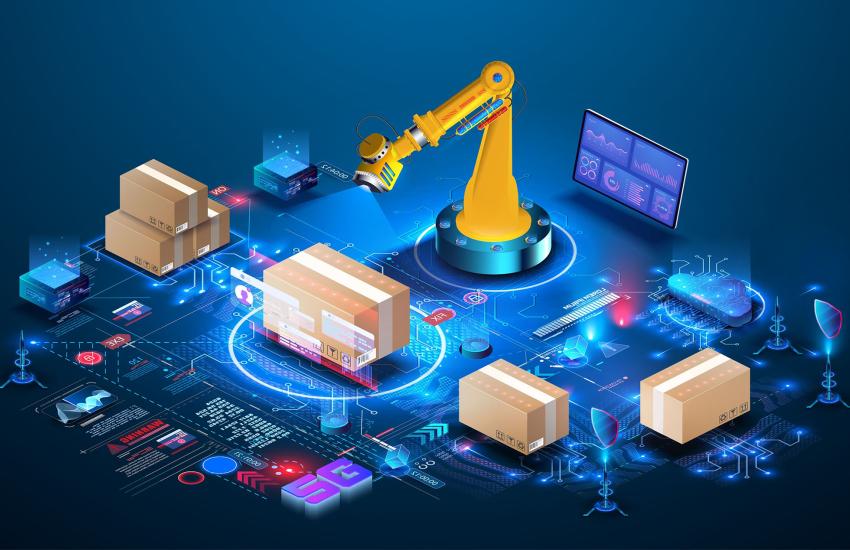