DARPA Envisions Commercial Uses for Autonomous Off-Road Vehicles
Autonomous, off-road vehicles being developed under the Defense Advanced Research Projects Agency’s (DARPA’s) Robotic Autonomy in Complex Environments with Resiliency (RACER) program may be capable of fulfilling a range of nontactical missions.
The DARPA program is developing new autonomy algorithm technologies—rather than vehicle or sensor technologies—that enable robotic ground vehicles to maneuver through off-road terrain at speeds high enough to keep up with manned military vehicles. Although only about halfway through the program, the DARPA team already is talking to officials in the Army and Marine Corps about the possibility of transitioning the technology to a program of record.
Possible programs include the Army’s Next Generation Combat Vehicle, which envisions teams of manned and unmanned armored vehicles working together, and the Navy Marine Expeditionary Ship Interdiction System (NMESIS), which will be the service’s first ground-based anti-ship missile capability. NMESIS combines the Kongsberg Defence & Aerospace anti-ship Naval Strike Missile with Raytheon’s Remotely Operated Ground Unit for Expeditionary (ROGUE) Fires Vehicle, which is built on the chassis of an Oshkosh Defense joint light tactical vehicle.
And the RACER technology might be useful for a plethora of commercial uses as well, according to Stuart Young, DARPA’s RACER program manager. “There are a lot of opportunities in construction and inspection in very remote areas. There are opportunities for logistics. In much more difficult terrains and areas that have no roads and structure, you can imagine opportunities in search and rescue, especially after a disaster,” Young said.
Aerial drones have proven useful in some of those missions, such as search and rescue, but have their limits. “Drones are great, but they have certain limiting endurance features. They can’t stay up for a long period of time. By nature of doing this on the ground, you can have much more persistence in an environment,” the program manager asserted. “You can imagine the oil and gas industry using this—farming, ranching, mining. We think there are those out there that are going to need capabilities beyond what they’re going to get from the specific self-driving industry.”
Although off-road vehicles do not have to deal with heavy traffic, pedestrians and other obstacles associated with urban areas, they do present unique challenges. On-road autonomy algorithms operate in well-structured and highly predictable environments, but military off-road autonomy algorithms have lagged due to the challenging complexity of off-road terrain environments and the need to travel at mission-relevant speeds, according to DARPA’s RACER webpage.
In an interview with SIGNAL Media, Young explained the challenges of off-road autonomous navigation. “If you can think about a self-driving car or self-driving vehicle, it’s typically driving on a road, which has a well-known and understood trafficability. We have to perceive the environment. And we have to reason about that terrain right in front of us. And sometimes a little bit farther in front of us, there’s a lot of uncertainty because we’re occluded from other objects in the environment.”
In such cases, the vehicle’s ability to rapidly process information about its surroundings becomes critical. “Processing that really fast is what has been a barrier to being able to go at speeds that are mission-relevant. We’ve been putting a lot of effort into understanding the scene and then reasoning about the scene. And then of course, you develop a plan on how you want to have the vehicle tackle that terrain,” Young elaborated. “Although we can handle trails, we’re talking truly off-road, reasoning about the terrain, bushes, negative obstacles, water features, ditches, rocks, trees, grasses, all those types of things are what makes it challenging.”
The lack of structure for off-roading presents another challenge. “We’re not taking advantage of any structure because there is none. We do all of our sensing onboard the vehicle because of that. And so that’s what makes the problem super challenging—having to do all that very fast is required for us to go at speeds that we’re trying to meet in RACER.”
RACER vehicles also will face an array of combat-related obstacles, such as destroyed vehicles and other debris. “Positive obstacles, like a bombed-out vehicle, are pretty easy for us to deal with; we can just route around it. It’s not all about avoiding. Those are also opportunities for us to exhibit tactical behaviors,” Young said. He added that the team will focus more on those tactical behaviors as the program progresses, including the potential for multiple vehicles working together.
He cited another example of unique obstacles the vehicles might face. “Where I just was, there are barbed wire fences. We don’t have a semantic class for barbed wire fences, but clearly, if you drive into a barbed wire fence, it’s not going to be the best.”
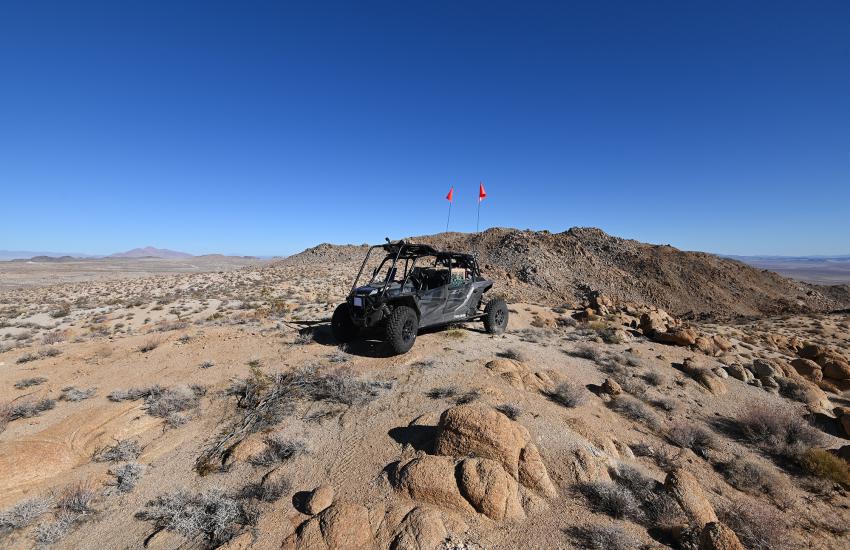
Ultimately, RACER’s artificial intelligence may be capable of identifying and avoiding enemy vehicles. “The caveats are that we’re not focused on that right this minute because the vehicle versus the environment is your base skill that you have to be able to do,” Young noted, describing the ability to detect and avoid enemy forces as a “next-level skill” that typically would “require different sensing and different algorithms.”
The initial goal is to build autonomous vehicles capable of running at least as fast as an M1 Abrams tank. A tank’s speed can vary quite a bit depending on the terrain, but Young estimates an average of about 28 kilometers (17.39 miles] per hour. “And then on trails, for example, they can go much faster, and we need to be able to go faster on those trails as well. That’s how we came up with the metrics on what we want to achieve.”
The four-year program consists of two phases, each about 24 months long. The first phase concluded in March, and the second was expected to begin in October. The teams for round one were led by Carnegie Mellon University, NASA-Jet Propulsion Laboratory, and the University of Washington.
The second phase will involve the approximately 9-ton Textron M5 vehicles with flat-top decks along with round one’s Polaris high-performance, all-terrain vehicles outfitted with world-class sensing and computational abilities. RACER technologies will apply to the full fleet of military ground vehicles, including those with wheels and tracks.
Also, in the final phase, DARPA will introduce “global planning and tactics,” which involve faster speeds, longer distances and more information, such as maps, fed to the vehicles. The goal is to see what happens when the robots are given a little more information and longer distances to travel while having to read and interpret the map, not only to traverse to a new location, but also, for example, to be more tactical and avoid silhouetting themselves on a hill crest.
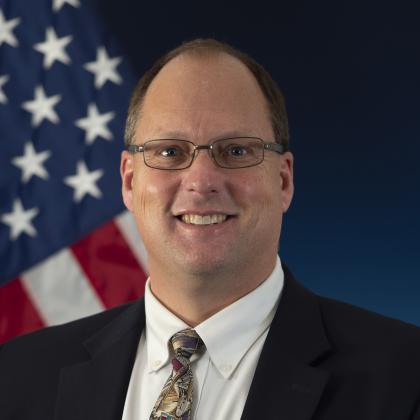
If you can think about a self-driving car, or self-driving vehicle, it’s typically driving on a road, which has a well-known and understood trafficability. We have to perceive the environment. And we have to reason about that terrain right in front of us.
Integrating maps also poses a challenge since off-road maps are not as detailed as city maps and will likely not be up to date during combat operations. “The terrain that you have ahead of time might be different—things destroyed or vehicles blocking your path. And you also don’t have a lot of information in environments under triple canopy, for example,” Young offered.
He added that RACER’s maps may include high-level information, such as mountains. “We found in a lot of our environments, the maps—even highest resolution maps that we can get—are insufficient for us to be able to see detailed features in the environment. But yes, there are opportunities for incorporating additional information like maps, and we’re growing into that.”
The program uses a variety of artificial intelligence technologies. “Pretty much everything that is available we’re using in some form or fashion. On the front end, we do a lot on the perception side. We use deep learning approaches. But we don’t have enough data to do that component exclusively, so we also incorporate self-supervised learning approaches where the robots can help train themselves,” Young reported. “And we’re also using inverse reinforcement learning.”
He added that those are the primary forms of artificial intelligence being incorporated but the team may explore others. “We don’t have the luxury of the amount of data that the self-driving community has because our state space is too big. We’ll never have enough, so we have to come up with approaches that are resilient—not because we fed them more data, but because the organic structure and architecture of the systems can handle things that are off-nominal.”
The program has collected less data than initially expected. “It’s not only about the volume of data, but it’s about the differences in the data for whatever skill you’re trying to do, whether it’s perceiving the environment, or whether you’re trying to control the vehicle,” Young said.
The teams will be given less data to work with in the second phase, Young reported. “In fact, at this next experiment, we’re not giving them any data of the actual location. They know where we’re going, but they don’t have any data of the actual environment. We want to learn how fast we can update our models and update our system as we enter a new environment.”
The teams are never given data about the actual test courses. “Similar to the way most machine learning approaches work, we give them similar data, and then obviously, we hold out our test areas for the actual tests. We will probably be doing a lot less data collection a priori, and that’s important because we are trying to have the robot be more resilient. In order to do that, we need the robots to have the capability to generalize a little bit better, so that’s part of the data strategy,” Young elaborated.
Comments