Data at the Edge: Navigating the Navy’s AI Revolution
Kenny Rogers’ description of a gambler, knowing what to throw away, knowing what to keep, could well apply to U.S. Navy data.
“As you’re producing a large vast amount of data, it’s about the ability to shed the right data that’s no longer desirable and retain the data that is still desirable,” said Capt. Jesse Black, commanding officer of the U.S. Naval Research Laboratory (USNRL).
All Navy assets produce increasing amounts of data, but to operate unmanned platforms there needs to be very large sets to adequately train artificial intelligence (AI) models to operate its systems.
“The ability to sense is gone through the roof. It’s cheap, cheap, and it’s so easy to sense now. The level of fidelity is huge,” Capt. Black said.
Founded in 1923, the USNRL is the corporate research laboratory for the Navy and the Marine Corps. It conducts a broad program of scientific research and technology development. Its work on AI, machine learning and unmanned platforms is part of the effort to multiply the Navy’s force.
Sensing is no longer a challenge. What is difficult is to bring the right amount of data with the correct system to act, including—or excluding—human intervention.
“Finding that right sweet spot of what’s necessary,” Capt. Black said.
An evolving occurrence sensed by the system should be compared to training and predetermined conditions, and homeostasis measures must be implemented. Still, all these processes must be programmed to avoid surprises for operators.
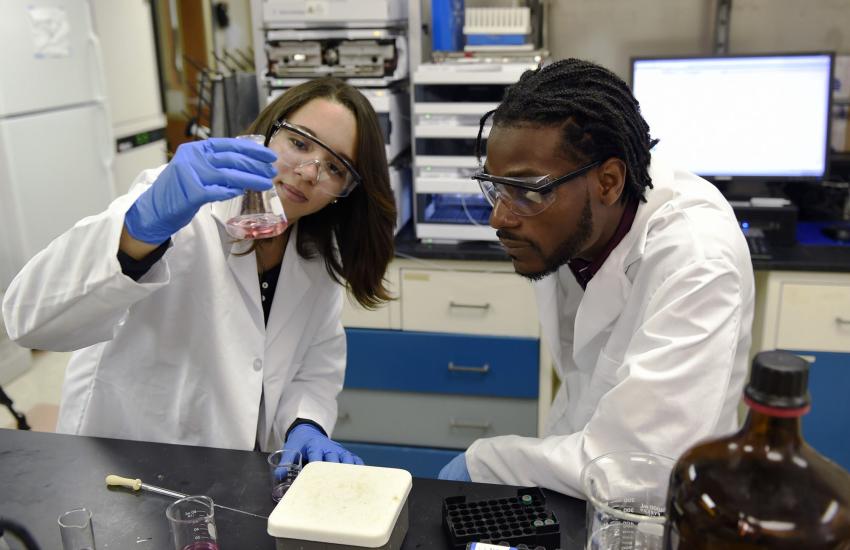
Regardless of the quality of the systems and the engineering behind them, it is not easy to replace warfighters with AI systems.
“A sailor may get a very wide range of data points for a variety of different ships, a variety of different classes in a variety of different locations and be generally an expert in everything. Whereas this machine learning algorithm may very quickly get just this one database, and it’s very constrained to just ships from this particular part of the world, this particular organization that you’re trying to better understand, et cetera,” David Aha, director, Navy Center for Applied Research in AI at USNRL, explained.
Training a system to have the flexibility and cognition of an experienced warfighter is not only about ingesting more data, and these abilities are better employed by a human brain.
“There’s still a lot more work that needs to get done,” Capt. Black said.
No amount of research or budget will create an immediate result. This also applies to AI and its related capabilities, like unmanned devices. And Black compared the honing of an algorithm with the time it takes to build the skills of a naval officer.
“It takes time for the learning process to happen, and so I think that same sort of evolution happens as we watch these algorithms, and the application of what we do—it takes time to develop them and mature them and grow them,” Capt. Black said.
This visible growth in algorithms’ capabilities helps build not only a more refined AI but also trust in its various use cases.
Another component of trust is being able to explain how a system arrived at a specific outcome.
Selecting the right tool is another key to success.
“If it’s sonar, sound, imagery, voice, something of that nature, then I might be thinking I’ve got to have some role of a deep net that can work with that kind of modality. If not, then I may be better off using some more traditional techniques, decision tree, induction rule, induction algorithms, you name it,” Aha said.
And the best way to optimize data, hardware and capability is through evolution, not necessarily more complexity.
“Oftentimes, deep learning systems, they will be able to sift in order to be able to identify patterns of interest, but they might have done so with one-millionth of the amount of data that we provided to it. We just don’t necessarily know that at the time,” Aha said.
Making something more efficient allows it closer to the edge, where processing capacity and communications can be restricted. Consequently, this brings more capabilities to forward deployed warfighters.
“We can place this on an edge device such that before we didn’t know how we were going to be able to provide it, because we wouldn’t be able to provide enough storage on that, but now we can because we know what model is effective for making those kinds of decisions for us, predictions for us.” Aha added.
The edge is a risky place, though. An adversary can access a device, and deciding how much computing power and which algorithms could potentially fall into the hands of the enemy is a difficult balancing act.
For Capt. Black, this tension has dimensions that are best addressed with flexibility and he offered the example of smart cars. While much processing is done in the car, to avoid pedestrians, for example, the vehicle relays data back to its manufacturer to improve systems.
“It’s a harmonious condition that they both exist, and it’s time-dependent on when, where and why. You would need to be on one versus the other. And so I like to kind of demystify the debate we should be in both and leveraging both,” Black said.
Part of this balance also revolves around speed at the edge, with the right amount of data processed in accordance with power and capacity.
Aha pointed out that edge devices’ communications could be limited due to conditions set by the adversary or by the environment. Underwater communications are difficult and keeping devices connected in that environment is challenging, according to Aha.
Comments